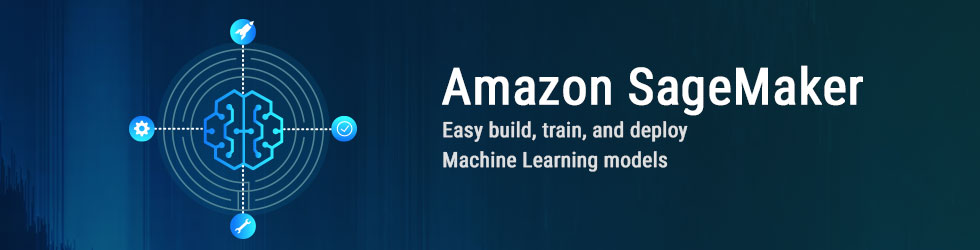
Machine Learning (ML) has become the talk of the town, and its usage has grown inherent in virtually all spheres of the technology sector. As more applications are beginning to employ the use of ML in their functioning, there is a tremendous possible value for businesses. However, developers have still had to overcome many obstacles to harness the power of ML in their organizations.
Keeping the difficulty of deployment in mind many developers are turning to Amazon Web Services (AWS). Some of the challenges to processing include correctly collecting, cleaning, and formatting the available data. Once the dataset is available, it needs to be prepared, which is one of the most significant roadblocks. Post processing, there are many other procedures which need to be followed before the data can be utilized.
Why should developers use the AWS Sagemaker?
Developers need to visualize, transform, and prepare their data, before drawing insights from it. What’s incredible is that even simple models need a lot of power and time to train. From choosing the appropriate algorithm to tuning the parameters to measuring the accuracy of the model, everything requires plenty of resources and time in the long run.
With the use of AWS Sagemaker, data scientists provide easy to build, train and use Machine learning models, which don’t require extensive training knowledge for deployment. Being an end-to-end machine learning service, Amazon’s Sagemaker has enabled users to accelerate their machine learning efforts, thereby allowing them to set up and install production applications efficiently.
Bid farewell to heavy lifting along with guesswork, when it comes to using machine learning techniques. Amazon’s Sagemaker is trained to provide easy to handle pre-built development notebooks, while up-scaling popular machine learning algorithms aimed at handling petabyte-scale datasets. Sagemaker further simplifies the training process, which translates into shorter model tuning time. In the expressions of the AWS experts, the idea behind Sagemaker was to remove complexities, while allowing developers to use the concepts of Machine Learning more extensively and efficiently.
Visualize and Explore Stored Data
Being a fully managed environment, it’s easier for Sagemaker to help developers visualizer and explore stored data. The information can be modified with all of the available popular libraries, frameworks, and interfaces. Sagemaker has been designed to include the ten most commonly used algorithm structures, some of which include K-means clustering, linear regression, principal component analysis and factorization machines. All of these algorithms are designed to run ten times faster than their usual routines, allowing processing to reach more efficient speeds.
Increased Accessibility for Developers
Amazon SageMaker has been geared to make training all the more accessible. Developers can just select the quantity and the type of Amazon EC2 instances, along with the location of their data. Once the data processing process begins within Sagemaker, a distributed compute cluster is set up, along with the training, as the output is setup and directed towards Amazon S3. Amazon SageMaker is prepared to fine-tune models with a hyper-parameter optimization option, which helps adjust different combinations of algorithms, allowing the developers to arrive at the most precise predictions.
Faster One-Click Deployment
As mentioned before, Sagemaker takes care of all launching instances, which are used for setting up HTTPS end-points. This way, the application achieves high throughput with a combination of low latency predictions. At the same time, it auto-scales various Amazon EC2 instances across different availability zones (AZ) to accelerate the processing speeds and results. The main idea is to eliminate the need for heavy lifting within machine learning so that developers don’t have to indulge in elaborate coding and program development.
Conclusion
Amazon’s Sagemaker services are changing the way data is stored, processed, and trained. With a variety of algorithms in place, developers can wet their hands with the various concepts of Machine Learning, allowing themselves to understand what goes on behind the scenes. All this can be achieved without becoming too involved in algorithm preparations and logic creation. An ideal solution for companies looking forward to helping their developers focus on drawing more analytics from tons of data.
Related Stories
Overcoming Cloud Security Threats with AI and Machine Learning
aws reinvent 2017 product announcements
5 exciting new database services from aws reinvent 2017