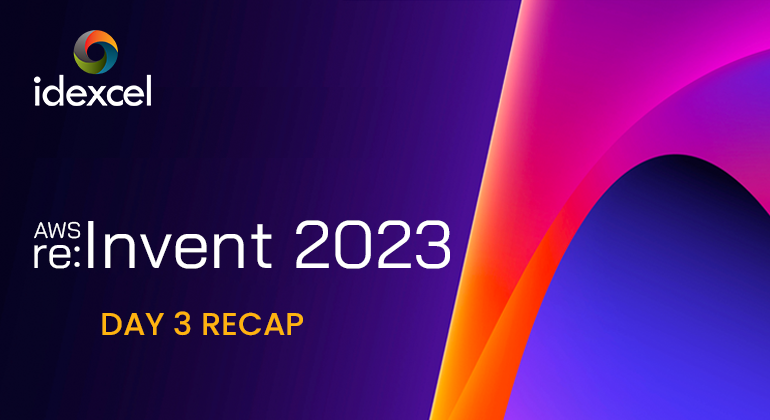
Amazon Neptune Analytics
Neptune Analytics, merging graph and vector database capabilities for enhanced insights in generative AI applications. The fully managed service enables users to analyze Neptune graph data or data lakes on Amazon S3, leveraging vector search for rapid discovery of key relationships. Swami Sivasubramanian, VP of Data and ML at AWS highlighted the synergy between graph analytics and vectors in uncovering hidden data relationships. Neptune Analytics streamlines infrastructure management, allowing users to focus on queries and workflows, automatically allocating resources based on graph size. The service is now available on a pay-as-you-go model in seven AWS regions.
Amazon SageMaker HyperPod
SageMaker HyperPod, a purpose-built service for training and fine-tuning large language models (LLMs), is now generally available. Optimized for distributed training, HyperPod creates a distributed cluster with accelerated instances, enabling efficient model and data distribution for accelerated training processes. The service allows users to save checkpoints, facilitating pause, analysis, and optimization without restarting. With fail-safe for GPU failures, HyperPod ensures a resilient training experience. Supporting Amazon’s Trainium and Trainium 2 chips or Nvidia-based GPU instances, including H100 processors, HyperPod can enhance training speeds by up to 40%, streamlining ML model development.
AWS Titan Image Generator
Titan Image Generator for content creators, allowing rapid creation and refinement of images through English natural language prompts. Catering to advertising, e-commerce, and media sectors, the tool produces studio-quality, realistic images at scale and low cost. It facilitates iterative image concept development by generating multiple options based on text descriptions and comprehending complex prompts with diverse objects. Trained on high-quality data, it ensures accurate outputs, featuring inclusive attributes and minimal distortions. The Titan Image Generator incorporates image editing functionalities, supporting automatic edits, inpainting, and outpainting. Users can customize the model with proprietary data, maintaining brand consistency, and it includes safeguards against harmful content generation, embedding invisible watermarks to identify AI-generated images, and discouraging misinformation.
Amazon OpenSearch Service zero-ETL integration with Amazon S3
Amazon unveils the customer preview of Amazon OpenSearch Service zero-ETL integration with Amazon S3, streamlining operational log queries in S3 and data lakes. This eliminates the need for tool-switching while analyzing operational data, enhancing query performance, and enabling the creation of fast-loading dashboards. Users leveraging OpenSearch Service and storing log data in Amazon S3 can now seamlessly analyze and correlate data across sources without costly and complex data replication. The integration simplifies complex queries and visualizations, offering a more efficient approach to understanding data, identifying anomalies, and detecting potential threats without the necessity of data movement.
Amazon Redshift now supports Multi-AZ (Preview) for RA3 clusters
Amazon Redshift is introducing Multi-AZ deployments that support running your data warehouse in multiple AWS Availability Zones (AZ) simultaneously and continue operating in unforeseen failure scenarios. A Multi-AZ deployment is intended for customers with business- critical analytics applications that require the highest levels of availability and resiliency to AZ failures. A Redshift Multi-AZ deployment allows you to recover in case of AZ failures without any user intervention. A Redshift Multi-AZ deployment is accessed as a single data warehouse with one endpoint and helps you maximize your data warehouse performance by distributing workload processing across multiple AZs automatically. You are not required to make any application changes to maintain business continuity during unforeseen outages.
Amazon SageMaker Canvas
SageMaker Canvas now supports foundation model-powered natural language instructions for data exploration, analysis, visualization, and transformation. This feature, powered by Amazon Bedrock, allows users to explore and transform their data to build accurate machine-learning models. Data preparation is often the most time-consuming part of the ML workflow, but SageMaker Canvas offers 300+ built-in transforms, analyses, and in-depth data quality insights reports without writing any code (like an example the quality of my dataset to identify any outliers or anomalies, we can make SageMaker Canvas to generate a data quality report to accomplish this task.). The process of data exploration and preparation is faster and simpler with natural language instructions, queries, and responses, allowing users to quickly understand and explore their data.
Schedule a meeting with our AWS cloud solution experts and accelerate your cloud journey with Idexcel.